Next: Adaptive filtering from the
Up: Noise reduction from the
Previous: The Wiener-like filtering in
Hierarchical Wiener filtering
In the above process, we do not use the information between the wavelet
coefficients at different scales. We modify the previous
algorithm by introducing a prediction wh of the wavelet coefficient from
the upper scale. This prediction could be determined from the regression
[2] between the two scales but better results are obtained
when we only set wh to Wi+1. Between the expectation
coefficient Wi and the prediction, a dispersion exists where we
assume that it is a Gaussian distribution:
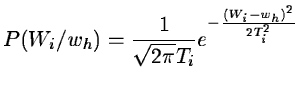 |
|
|
(14.84) |
The relation which gives the coefficient Wi knowing wi and wh is:
 |
|
|
(14.85) |
with:
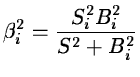 |
|
|
(14.86) |
and:
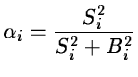 |
|
|
(14.87) |
This follows a Gaussian distribution with a mathematical expectation:
 |
|
|
(14.88) |
with:
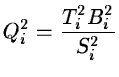 |
|
|
(14.89) |
Wi is the barycentre of the three values wi, wh, 0 with the
weights Ti2, Bi2, Qi2. The particular cases are:
- If the noise is large (
)
and even if the correlation
between the two scales is good (Ti is low), we get
.
- if
then
.
- if
then
.
- if
then
.
At each scale, by changing all the wavelet coefficients wi of the
plane by the estimate value Wi, we get a Hierarchical Wiener
Filter. The algorithm is:
- 1.
- Compute the wavelet transform of the data. We get wi.
- 2.
- Estimate the standard deviation of the noise B0 of the first plane
from the histogram of w0.
- 3.
- Set i to the index associated with the last plane: i = n
- 4.
- Estimate the standard deviation of the noise Bi from B0.
- 5.
-
Si2 = si2 - Bi2 where si2 is the variance of wi
- 6.
- Set wh to Wi+1 and compute the standard deviation Tiof wi - wh.
- 7.
-

- 8.
- i = i - 1. If i > 0 go to 4
- 9.
- Reconstruct the picture
Next: Adaptive filtering from the
Up: Noise reduction from the
Previous: The Wiener-like filtering in
Petra Nass
1999-06-15